July 25, 2022
Amazon Web Services (AWS) DeepRacer is a league where developers of all skill levels can train to compete against each other through machine learning. Competitors build models using reinforcement learning to compete virtually every month in the AWS DeepRacer League to qualify for a spot in the championship, where they can see their virtual model in a real-world, physical environment.
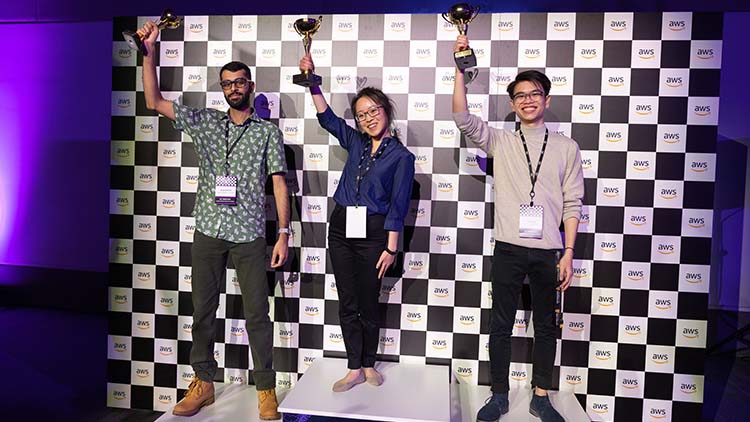
Data Science Intern Daphne recently competed at the AWS DeepRacer Canadian Wildcard Championship in Ottawa, and guess what! She won the whole thing!
AWS DeepRacer started in 2018 as the first autonomous racing league of its kind. There are two leagues within DeepRacer, a professional and student league. Daphne competed in the student league.
"I was there with 12 other undergraduate and graduate students selected because we had the best times in our respective provinces," she said. "You could say we were the best of the best.”
Daphne explained that most of the students competing were engineers. However, one business student was competing as well. "That shows how easy it is for developers of all skill levels to get started in machine-learning racing."
According to Daphne, about 200-300 people were spectators at the competition, including some Members of Parliament and AWS employees, to name a few.
Daphne describes the event as similar to a dog show. "You've trained your dog what to do, but sometimes the dog might not behave as expected. And that's exactly what we see with these cars."
Before arriving in Ottawa to compete, Daphne trained approximately 30 different models virtually. Then, working up to the championship, she made minor tweaks as she went to get a feel for what works and what doesn't.
Daphne said competitors had one day to get their testing and racing completed. "We had to be smart with how we wanted to use our time. In total, each of us had around eight minutes to test train and get acquainted with the differences between the virtual environment and the physical one."
"I had around five or six models that I thought would perform pretty well on that live track," she said. "But as life is always full of surprises, when I uploaded my models onto the car, most models couldn't even get the car to finish one continuous lap."
At this point in the competition, Daphne had to tap into her critical thinking skills. She broke down her overarching task into smaller chunks to make it more manageable. She wanted to win the competition, but the first step was to get the model to complete one continuous loop around the track.
"After I was able to get it to complete one continuous lap, I was able to obtain that fastest lap time by improving my models," she said.
Well, it worked for her. Daphne came in first place in the student competition, clocking just over 11 seconds, just beating the second-place competitor by a little over a tenth of a second.
She described autonomous racing to be like teaching a baby to walk. Reinforcement learning allowed her to train her model to complete a loop on the track by learning to “maximize rewards.”
"After numerous iterations, it will eventually learn that if it just follows the optimal path, it can accumulate the largest total sum of rewards at the very end when it reaches that finish line. And that's how it learns to follow the behaviour we instructed it to figure out itself."
She emphasized the importance of simplicity when coding. "Sometimes it's not about complexity that's the most important aspect, but it's simplicity, because when the models become too complex, the cars will start to get confused about what we're trying to optimize."
She added that waypoints, which "are numbers along different intervals of the path that can help to make your training more customizable," are significant in training your autonomous car.
For example, if your model needs to follow an "S" curve, you can modify the speed to three to five seconds faster by cutting corners. "Customizing my waypoints helped me train my winning model."
After her big win in Ottawa, Daphne and a few friends are starting an autonomous racing league at the University of Calgary! They have big plans to expand to Western Canada and hopefully all across the country.
She said they are still in the early stages of planning, but the Schulich School of Engineering and AWS are supporting the group! Schulich signed on to be an official sponsor!
"We hope that we'll be able to enable and empower more individuals to learn about the applications of machine learning by offering some workshops that will go over the fundamentals of reinforcement learning."
Sounds excellent, Daphne! We can't wait to learn more!
Use the links below to share this article on LinkedIn, Twitter, Facebook, or via email.